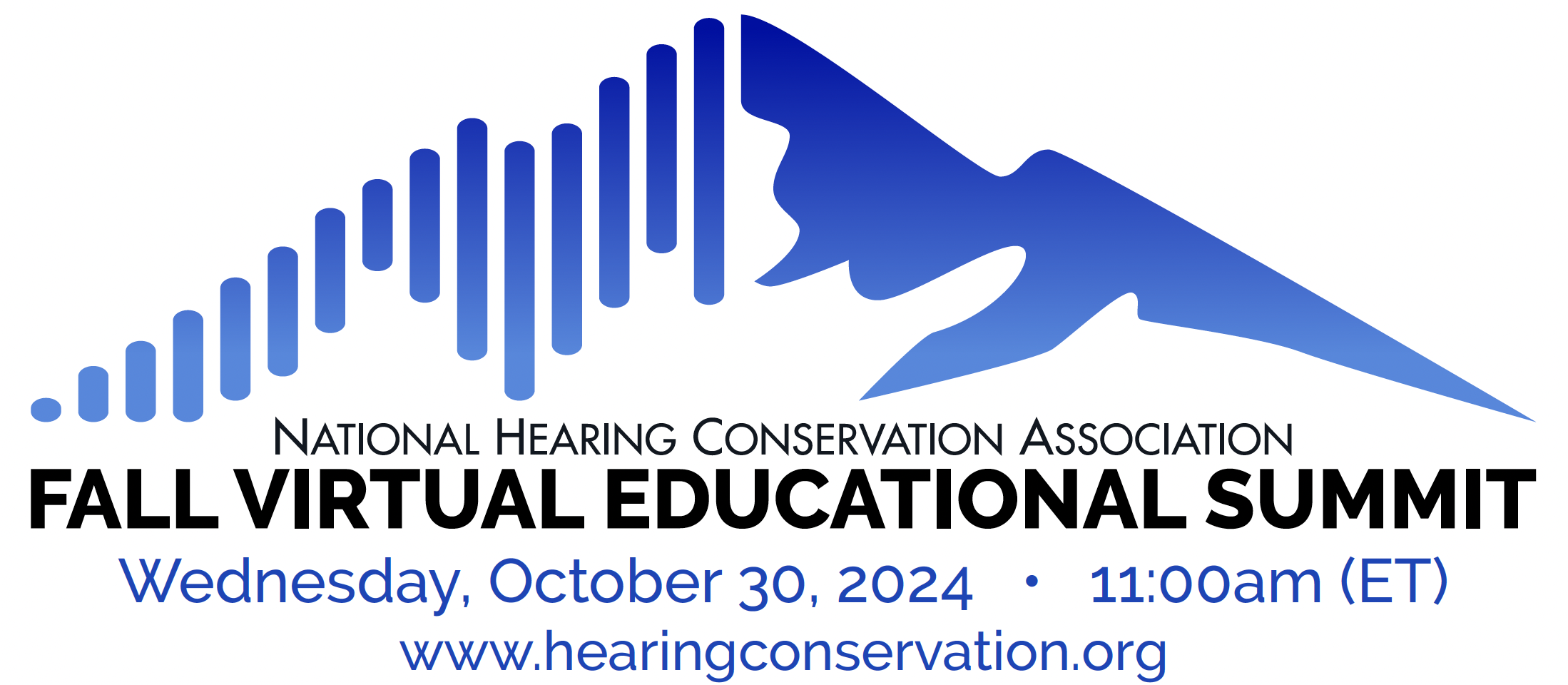
Drowning in Data: Opportunities and Challenges in Assessing Noise Exposure
Speaker: Benjamin Roberts, MPH, PhD, CIH Organization: Benchmark Risk Group Job Title: Supervising Risk Scientist
This session covers data sources and best practices for managing large noise exposure datasets. It will also explore common statistical tools for analyzing data and how advancements in machine learning and AI are creating new opportunities for understanding noise exposure.
- Learning Objective 1: Learners will be able to identify and explain different sources of noise exposure data in audiology, including their benefits, drawbacks, and applications
- Learning Objective 2: Learners will be able to demonstrate best practices for managing and organizing large noise exposure datasets, with a focus on improving data handling efficiency in audiology.
- Learning Objective 3: Learners will gain familiarity with statistical tools and machine learning techniques to analyze noise exposure data, and how these tools can enhance data interpretation and understanding.
About Benjamin
Dr. Benjamin Roberts is a Supervising Risk Scientist at Benchmark Risk Group. He completed a Ph.D. in Environmental Health Science and an MPH in industrial hygiene, both from the University of Michigan. He is also a Certified Industrial Hygienist. His research is focused on the statistical analysis of occupational and environmental exposure data with an emphasis on using new technology and rigorous statistical methods to improve decision-making. Dr. Roberts is a member of the American Industrial Hygiene Association (AIHA) and is active in the Big Data and Sensors Technology Content Portfolio Advisory Group (CPAG) and is also the past chair of the AIHA Noise Committee. He is also an adjunct faculty member at Grand Valley State University (GVSU) in Grand Rapids, MI. He is currently an expert panel member of the Safe In Sound committee. He has authored or co-authored two book chapters, one monograph for the WHO, and 23 peer-reviewed manuscripts and has presented at several conferences on the topics of noise, silica, and asbestos exposure, frequentist and Bayesian statistical analysis, and big data. During his doctoral work, he partnered with the NIOSH to develop laboratory and field studies to evaluate the efficacy of measuring occupational noise exposure using smart devices. He also utilized approximately one-million occupational noise measurements to develop a job-exposure matrix (JEM) for noise. Finally, he performed a longitudinal analysis of noise exposure and hearing loss in construction workers and identified the most optimal exposure metrics for predicting noise induced hearing loss.
Financial Disclosures: None Non-Financial Disclosures: None Click here for Speaker Conflict of Interest Forms
|